Academia, like many other sectors, is a complex work environment. Although universities vary in terms of their size and objectives, the average university in the United States, Canada, UK, and EU must simultaneously serve the interests of undergraduate education, graduate education, professional education, basic research, applied research, public policy research, and basic scholarship. Most research universities receive funding for operation from a combination of public and private sources. For example, my home university, The University of Western Ontario, receives its operating funds from tuition payments, governments, research funding agencies, and from private donors. Many other research universities are funded in similar ways, and most smaller colleges are as well.
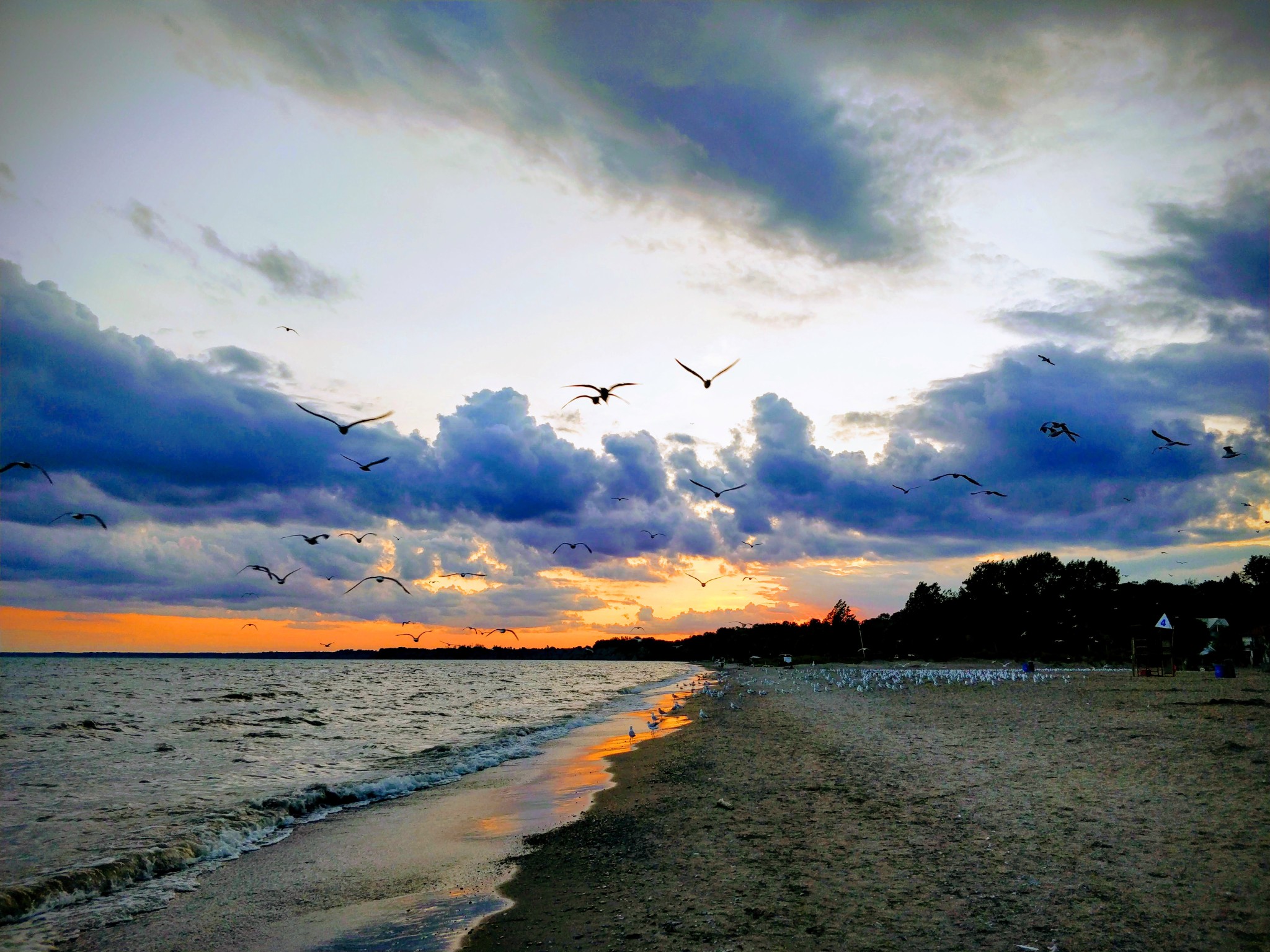
Looking west over Lake Erie, Port Stanley, Ontario
Faculty are at the center of this diverse institution, acting as the engine of teaching, research, and service. As a result, faculty members may find themselves occasionally struggling to manage these different interests. This article looks at the challenges that faculty members face, paying particular attention to the leadership role that many faculty play. I then explore the possible ways in which a mindfulness practice can benefit faculty well-being and productivity.
Challenges of Leadership in the University Setting
Although many work environments have similar challenges and issues (being pulled in different directions, time management, etc.) I want to focus on the challenges that faculty members face when working at and leading the average, mid-sized or large university. The specific challenges will vary in terms of what role or roles a person is serving in, but let’s first look at challenges that might be common to most faculty members.
Challenge 1: Shifting tasks
“Email is a wonderful thing for people whose role in life is to be on top of things. But not for me; my role is to be on the bottom of things. What I do takes long hours of studying and uninterruptible concentration.” — Donald Knuth
I love this quote from Donald Knuth, a professor of computer science, because it encapsulates the main challenge that so many of us have. We want to be on top of things (teaching, questions from students, cutting-edge research) but we also want to be on the bottom: digging deeply into a problem and finding a solution.
The average faculty member has, at a minimum, 2–3 very different kinds of jobs. We’re teachers, researchers/scholars, and we also help to run the university. Within these broadly-defined categories, we divide our teaching time between graduate and undergraduate teaching and mentorship. Research involves investigation, applying for grants, reading, investigation, analysis, writing, dissemination. And running the university can make us managers, chairs, deans, and provosts and as such, we’re responsible for hiring research staff, hiring other faculty members, and managing budgets.
These three categories require different sets of skills and shifting between them can be a source of stress. In addition, the act of shifting between them will not always go smoothly and this may result in a loss of effectiveness and productivity as the concerns from one category, task, or role bleed into another. Being mindful of the demands of the current task at hand is crucial.
For example, I find it especially difficult to transition after 2–3 hours of leading a seminar or lecture. Ideally, I would like to have some time to unwind. But many times, I also need to schedule a meeting in the afternoon and find that I have only a short amount of time to go from “lecture mode” into “meeting mode”. Worse, I might still be thinking about my lecture when the meeting begins (this is an even bigger challenge for me in 2020, because nearly everything is online, on Zoom, from my home office). Even among university leaders that have little or no direct teaching requirements, it is common to have to switch from and to very different topics. One day you might start the day answering emails (with multiple topics), a morning meeting on hiring negotiations, a meeting about undergraduate planning, then an hour with a PhD student on a very specific and complex analysis of data for their dissertation research, followed by a phone call from the national news outlet asking about the research of one of your faculty members. Shifting between these tasks can reduce your effectiveness. The cognitive psychology literature refers to this as “set shifting” or “task-shifting”, and research has supported the idea that there is always a cost to shift (Arrington & Logan, 2004; Monsell, 2003). These costs will eventually affect how well you do your job and also how you deal with stress. It’s difficult to turn your full attention to helping your student with an analysis when you are also thinking about your department’s budget.
As academics, we switch and shift tasks throughout the day and throughout the week. The primary challenge in this area is to be able to work on the task at hand and to be mindful of distractions. Of course, they will occur, but through practice, it may be possible to both minimize their impact and also reduce the stress and anxiety associated with the distractions.
Challenge 2: Shared governance
One aspect of academia that sets it apart from many corporate environments is the notion of “shared governance”. Though this term is common (and has been criticized as being somewhat empty,) the general concept is that a university derives its authority from a governing board, but that faculty are also vested in the institutional decision-making process. This means that most universities have a faculty senate that sets academy policy, dean’s level committees that review budgets and programs, and departmental committees that make decisions about promotion and tenure, hiring, and course assignments.
From a leadership perspective, this can mean that as a chair or dean you are always managing personal, balancing the needs of faculty, students, budgets, senior administrators, and the public image of your university. There may not be a clear answer to the question of “who is the boss?” Sometimes faculty are asked to assume leadership roles for a set time and will need to shift from a collegial relationship to a managerial one (then back to a collegial one) for the same people. That is, one day you are colleagues and the next you are his or her supervisor.
The challenge here is to understand that you may be manager, colleague, and friend at the same time. In this case, it’s very helpful to be mindful of how you interact with your colleagues such that your relationship aligns with the appropriate role.
Challenge 3: Finding time for research and scholarship
One of the most common complaints or concerns from faculty is that they wish they had more time for research. This is a challenge for faculty as well as leaders. Although a common workload assumes that a faculty member may spend 40% of their time on research, most faculty report spending much of their time in meetings. However, promotion and tenure is earned primarily through research productivity. Grants are awarded to research productive faculty. That is, most of those meetings are important, but do not lead to promotion and career advancement. This creates a conflict that can cause stress because although 40% is the nominal workload, it may not be enough to be research productive. Other aspects of the job, like meetings related to teaching and service, may take up more than their fair share but often feel more immediate.
In order to be effective, academic leaders also need to consider these concerns from different perspectives. For example, when I was serving as the department chair for a short period, I had to assigned teaching to our faculty. There are courses that have to be offered and teaching positions that have to be filled. And yet my colleagues still need to have time to do research and other service work. These can be competing goals and they affect different parts of the overall balance of the department. The department chair needs to balance the needs of faculty to have adequate time for research with the needs of the department to be able to offer the right amount of undergraduate teaching. So not only is it a challenge to find time to do one’s own research, a department chair also needs to consider the same for others. Being mindful of these concerns and how they come into conflict is an important aspect of university leadership.
Considering these diverse goals and trying to meet them requires a fair degree of cognitive flexibility and if you find yourself being pulled to think about teaching, about meetings, and about the workload of your colleagues, it is going to pull you away from being able to be on top of your own research and scholarship. The primary challenge in this area is to create the necessary cognitive space for thinking about research questions and working on research.
Mindfulness and Leadership
I’ve listed three challenges for leaders in an academic setting: switching, shared governance, and finding time for research. There are more, one course, but let’s stick with these. I want to now explain what mindfulness practice is and how it might be cultivated and helpful for academic leaders. That is, how can mindfulness help with these challenges?
What is mindfulness?
A good starting point for this question is a definition that comes from Jon Kabat-Zinn’s work. Mindfulness is an open and receptive attention to, and awareness of what is occurring in the present moment. For example, as I’m writing this article, I am mindful and aware of what I want to say. But I can also be aware of the sound of the office fan, aware of the time, aware that I am attending to this task and not some other task. I’m also aware that my attention will slip sometimes, and I think about some of the challenges I outlined above. Being mindful means acknowledging this wandering of attention and being aware of the slips but not being critical or judgmental about my occasional wavering. Mindfulness can be defined as a trait or a state. When described as a state, mindfulness is something that is cultivated via mindfulness practice and meditation.
How can mindfulness be practiced?
The best way to practice mindfulness is just to begin. Mindfulness can be practiced alone, at home, with a group, or on a meditation retreat. More than likely, your college or university offers drop in meditation sessions (as mine does). There are usually meditation groups that meet in local gyms and community centers. Or, if you are technologically inclined, the Canadian company Interaxon makes a small, portable EEG headband called MUSE that can help develop mindfulness practice (www.choosemuse.com). There are also excellent apps for smartphones, like Insight Timer.
The basic practice is one of developing attentional control and awareness by practicing mindfulness meditation. Many people begin with breathing-focused meditation in which you sit (in a chair or on a cushion) close your eyes, relax your shoulders and concentrate on your breath. Your breath is always there, and so you can readily notice how you breath in and out. You notice the moment where your in-breath stops and your out-breath begins. This is a basic and fundamental awareness of what is going on right now. The reason many people start with breathing-focused meditation is that when you notice that your mind begins to wander, you can pull your attention back to your breath. The pulling back is the subtle control that comes from awareness and this is at the heart of the practice. The skill you are developing with mindfulness practice is the ability to notice when your attention has wandered, not to judge that wandering, and to shift your focus back to what is happening in the present
Benefits of mindfulness to academic leaders
A primary benefit of mindfulness involves learning to be cognitively and emotionally present in the task at hand. This can help with task switching. For example, when you are meeting with a student, being mindful could mean that you bring your attention back to the topic of the meeting (rather than thinking about a paper you have been working on). When you are working on a manuscript, being mindful could mean keeping your attention on the topic of the paragraph and bringing it back from other competing interests. As a researcher and a scientist, there are also benefits as keeping an open mind about collected data and evidence which can help to avoid cognitive pitfalls. In medicine, as well as other fields, this is often taught explicitly as at the “default interventionist” approach in which the decision-maker strives to maintain awareness of her or her assessments and the available evidence in order to avoid heuristic errors. (Tversky & Kahneman, 1974) As a chair or a dean, being fully present could also manifest itself by learning to listen to ideas from many different faculty members and from students who are involved in the shared governance of academia.
Cognitive and clinical psychological research has generally supported the idea that both trait mindfulness and mindfulness meditation are associated with improved performance on several cognitive tasks that underlie the aforementioned challenges to academic leaders. For example, research studies have shown benefits to attention, working memory, cognitive flexibility, and affect. (Chambers, Lo, & Allen, 2008; Greenberg, Reiner, & Meiran, 2012; Amishi P. Jha, Stanley, Kiyonaga, Wong, & Gelfand, 2010; Amism P. Jha, Krompinger, & Baime, 2007) And there have been noted benefits to emotional well-being and behaviour in the workplace as well. This work has shown benefits like stress reduction, a reduction to emotional exhaustion, and increased job satisfaction (Hülsheger, Alberts, Feinholdt, & Lang, 2013, Nadler, Carswell, & Minda, 2020)
Given these associated benefits, mindfulness meditation has the potential to facilitate academic leadership by reducing some of what can hurt good leadership (stress, switching costs, cognitive fatigue) and facilitating what might help (improvements in attentional control and better engagement with others).
Conclusions
As I mentioned at the outset, I wrote this article from the perspective of a faculty member at large research university, but I think the ideas apply to higher education roles in general. But it’s important to remember that mindfulness is not a panacea or a secret weapon. Mindfulness will not make you a better leader, a better teacher, a better scholar, or a better scientist. Mindful leaders may not always be the best leaders.
But the practice of mindfulness and the cultivation of a mindful state has been shown to reduce stress and improve some basic cognitive tasks that contribute to effective leadership. I find mindfulness meditation to be an important part of my day and an important part of my role as a professor, a teacher, a scientist, and an academic leader. I think it can be an important part of a person’s work and life.
References
Arrington, C. M., & Logan, G. D. (2004). The cost of a voluntary task switch. Psychological Science, 15(9), 610–615.
Chambers, R., Lo, B. C. Y., & Allen, N. B. (2008). The Impact of Intensive Mindfulness Training on Attentional Control, Cognitive Style, and Affect. Cognitive Therapy and Research, 32(3), 303–322.
Greenberg, J., Reiner, K., & Meiran, N. (2012). “Mind the Trap”: Mindfulness Practice Reduces Cognitive Rigidity. PloS One, 7(5), e36206.
Hülsheger, U. R., Alberts, H. J. E. M., Feinholdt, A., & Lang, J. W. B. (2013). Benefits of mindfulness at work: the role of mindfulness in emotion regulation, emotional exhaustion, and job satisfaction. The Journal of Applied Psychology, 98(2), 310–325.
Jha, A. P., Krompinger, J., & Baime, M. J. (2007). Mindfulness training modifies subsystems of attention. Cognitive, Affective & Behavioral Neuroscience, 7(2), 109–119.
Jha, A. P., Stanley, E. A., Kiyonaga, A., Wong, L., & Gelfand, L. (2010). Examining the protective effects of mindfulness training on working memory capacity and affective experience. Emotion , 10(1), 54–64.
Monsell, S. (2003). Task switching. Trends in Cognitive Sciences, 7(3), 134–140.
Nadler, R., Carswell, J. J., & Minda, J. P. (2020). Online Mindfulness Training Increases Well-Being, Trait Emotional Intelligence, and Workplace Competency Ratings: A Randomized Waitlist-Controlled Trial. Frontiers in Psychology, 11, 255.
Tversky, A., & Kahneman, D. (1974). Judgment under Uncertainty: Heuristics and Biases. Science, 185(4157), 1124–1131.